There are over 2 million cooling towers in North America alone. They’re even more common than elevators, and building visitors feel their impact though they rarely see them. Cooling towers enable air and water to come together - using evaporation to remove heat as the people in the building create it.
However, cooling towers are often not well-adapted to local conditions and do not generally change operating modes in response to weather or seasons. These inefficiencies often thwart the plans of sustainability directors looking to reduce their building's carbon footprint and executives managing utility bill budgets. We have built automated machine learning systems that use live sensor data to recommend new cooling tower settings regularly, allowing them to operate more efficiently and thus save water, energy, and money.
Cooling Tower Operation
A cooling tower is a type of machine that can be found on many large buildings and is deceptively simple in appearance: often, it is just a big metal box with a fan inside and warm water running through it. Yet, cooling towers constitute a crucial component in many large cooling systems and are thus essential for cooling your apartment or office on a hot day.
A cooling tower typically does not have many settings, and often a building manager will buy and install one, maybe clean some leaves out a couple of times per year, but otherwise not modify its behavior for years at a time. The systems are reliable enough to make this possible, but our research has shown that this is often not the best way to operate them.
At Tagup, we’ve built an automated software platform that enables dynamic changes in equipment operation in response to changes in weather and load. We monitor chillers, pumps, and towers, and combine real-time performance data with theoretical thermodynamic models to minimize the energy it takes to power chiller plants.
Our machine-learning technology controls cooling towers so that the total cooling load is minimized, and the energy saved can be used to run the entire cooling system. Tagup technology lives in a constant state of re-training and finetuning, adapting to performance degradation of equipment with automated ease.
This has two related outcomes that benefit all involved: operating costs for buildings are reliably reduced, and the water and energy saved will help the environment. How cool is that?
Cooling a Building
If you live in an apartment building or work in a large office, then there is a good chance that the HVAC (Heating, Ventilation, and Air Conditioning) system has two components that allow it to keep you from sweating during a hot day: a chiller and a cooling tower. The chiller works in a similar way to how your fridge keeps your groceries cool: it compresses and expands a gas in a way that takes heat from one place and moves it to another. Your fridge moves the heat from the inside to its outside (which is why the back is warm!) while an HVAC chiller typically cools water running through it.
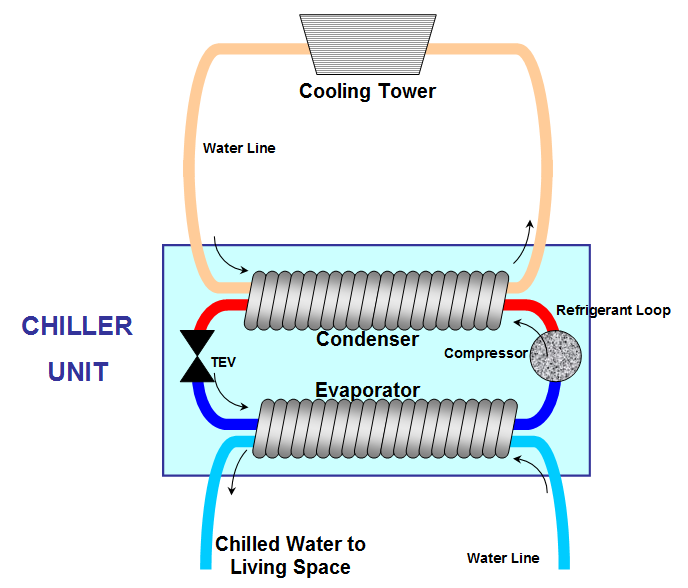
The chilled water is then circulated through heat exchangers that cool down air and blow it through ducts to cool the rooms. Since such a chiller will emit far more heat than your fridge, it needs another system that safely removes the heat to somewhere well away from the inside of a room. It does so by using another water circulation loop that sends hot water to a cooling tower (see diagram).
The cooling tower does not work like a fridge but rather makes use of a different physics trick: cooling through evaporation! When you drop some alcohol-based cologne on your skin, you will immediately feel a chill: The alcohol easily evaporates, i.e. turns from a liquid into a gas, and absorbs energy, i.e. heat, in the process. The same effect is also the reason why you sweat: your body emits water that can evaporate and thus cool you down.
For commercial cooling towers, engineers have perfected the design to let water flow over a large surface area inside it and use a fan to quickly blow the water vapor away and suck in new, less humid air for continued cooling. The remaining water1 in the tower is now cooler and can be circulated back into the chiller.
Improving Cooling Tower Operation
In typical building applications, cooling system efficiency depends strongly on weather, seasons, and the number of people (or computers) in the building, which will affect both the water temperature coming from the chiller and the outside air conditions that determine evaporation behavior. This can lead to vastly different and sometimes very inefficient operations, for example when the cooling tower tries to cool down the water to a preset temperature that cannot be achieved.
Efficiency is a major concern since cooling systems consume an enormous amount of energy. In the US, space cooling of residential and commercial buildings in 2019 consumed 380 billion kWh2 , or 10% of the country’s total electricity consumption, equivalent to approximately 170 million tons of CO2 emissions3. For further context, one metric ton of CO2 would have the weight of 400 bricks and be as tall, long, and wide as a telephone pole.
Often, this energy demand is split 90% to 10% between the cooling system’s main components, the chiller, one or multiple pumps, and the tower. Following the status quo, most facilities run their tower as hard as possible. Using a fixed set point strategy, they set a temperature goal lower than what their tower can achieve, forcing it to run as hard as possible at all times. However, simply running the tower harder isn’t an ideal solution, and this is where Tagup shines.
Recent results showed a 10% total energy savings compared to a fixed setpoint and schedule-based controls. Adjusting fan speeds and redistributing the relative workload between the chiller and cooling tower made these savings possible.
Water conservation can also be a key driver for optimization, especially in regions with water scarcity, promising significant savings of this precious, and in some cases expensive, resource. Some regions of the world, such as the American west, have experienced drought-like conditions for so long that they’ve begun adapting to the conditions as a new normal. An estimated 55 million people globally are directly affected by droughts each year, but a shift from reactive crisis-based efforts to proactive sustainability efforts can help turn the tide.

Cooling towers are often sold “as-is”, without in situ tuning for a given building, location, or load profile. And furthermore, advanced thermodynamic modeling for HVAC systems exists but is often focused on individual components. Thus, it typically fails to address inefficiencies due to weather changes and the non-linear relationship between a chiller and a cooling tower.
A more informed optimization scheme that will monitor the full HVAC system, as well as air temperature and humidity, can better balance the behavior of the different components and offer significant reductions in energy, water, and total operating cost.
Many of the factors that impact cooling system cost efficiency vary, not only over time but also from place to place. This could include, for example, extreme differences in humidity between Massachusetts and Arizona or smaller variations in the rates for electricity and water. Having a system that quickly adjusts HVAC operation based on such local factors can avoid unnecessary costs.
Cost benefits and efficiency become even more clear when we look at changes from year to year. The ongoing climate crisis will lead to more extreme temperatures and a larger number of hot days. Making cooling systems more efficient will help to avoid a dangerous feedback process in which increased energy demand leads to more CO2 emission and accelerated global warming.
Leveraging Machine Learning
Tagup has developed commercial technology that has shown up to 20% savings on energy and water costs. We have demonstrated how sensors on a cooling tower can yield a wealth of data, which is the basis for our real-time optimization of temperature settings that directly affect fan speed, water low rates, and chiller power. Data-driven machine learning is a crucial tool for producing such quantitative recommendations. Instead of trying to construct a detailed, time-dependent thermal model of all the components, fluids, and gases, we use a combination of sensor data and simulations as input for our self-learning software models.
With enough training data, such a machine-learning model can predict the effects of changes in outside weather conditions and inside cooling demands on the operation and efficiency of the chiller and cooling tower. We can then simulate and optimize the cooling tower operation for a simple objective:
Find the best settings that lead to the lowest energy and water usage, and thus the lowest cost, at any time.
Interested in learning more? Book a demo today.
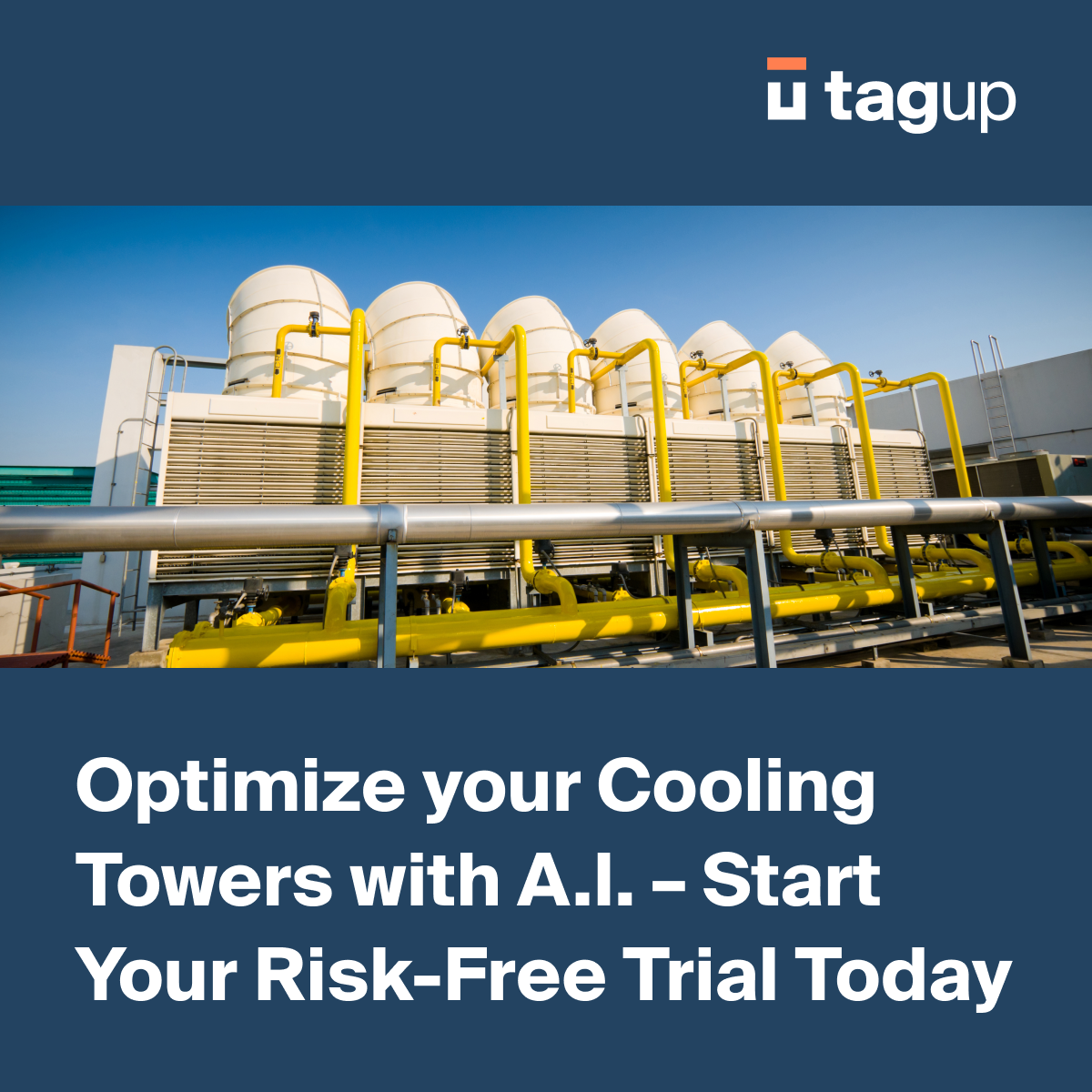
. . .
1 Sometimes, a different fluid in closed pipes or the air in the tower is the primary medium that is being cooled.
2 Data from U.S. Energy Information Administration: https://www.eia.gov/tools/faqs/faq.php?id=1174&t=3
3 Conversion based on U.S. Energy Information Administration: https://www.eia.gov/tools/faqs/faq.php?id=74&t=11