Hello and welcome to Utility Machine Learning!
The purpose of this blog is to share the latest developments in the application of data science and machine learning to the utilities space.
The blog is managed by Tagup Inc., a software and data science MIT spinout, headquartered in Somerville, Massachusetts. We develop machine learning models to automatically detect anomalous behavior and predict failures for a variety of equipment types.
While many of the blog posts will be authored by the data science team here at Tagup, we’ll also feature posts from utilities experts on how machine learning is used to create value and make principled decisions across the utility industry.
Machine learning encompasses the study and application of algorithms and statistical models to progressively improve performance on a specific task. During the past several decades, the field has flourished as computing power has increased exponentially and digital information became widely distributed via the Internet. Applying these models to problems within the utility sector can better model disruptive events, such as the malfunction or failure of an asset. With these prognostics, asset managers can perform maintenance actions or replace equipment before it fails. This pre-emptive action avoids service outages and potential damage resulting from catastrophic failures. The power of predictive analytics therefore enables utilities to reduce costs and the risks associated with unexpected equipment malfunctions.
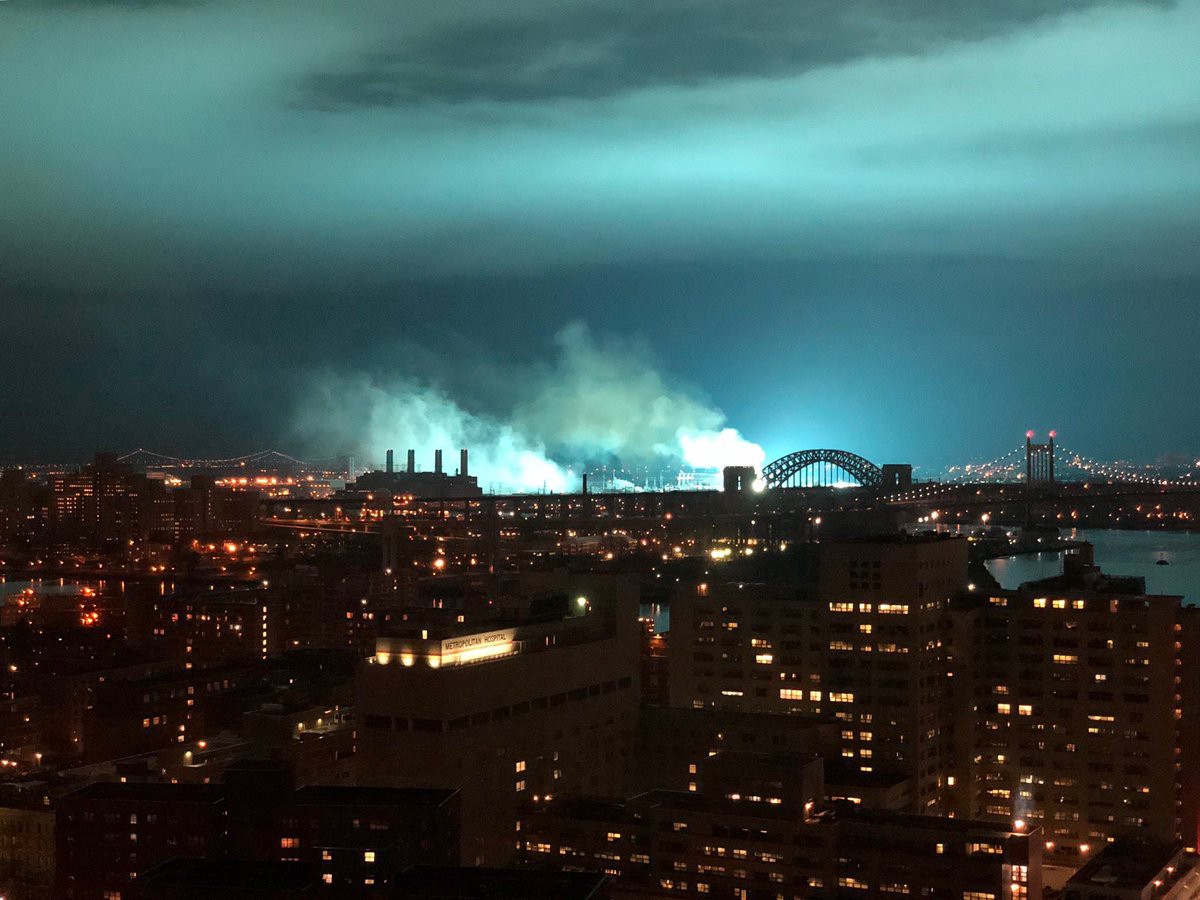
The focus of this blog is the application of predictive analytics and machine learning to improve asset management. We encourage submissions related to broader machine learning applications in the utility industry—including load forecasting, power flow modeling, and customer/outage analytics. The content is not intended solely for practitioners; our data science team will publish articles related to original research and novel application of data science techniques, of interest to data scientists working outside the utility industry.
We strive to make our content as accessible as possible to a variety of audiences with differing levels of technical background, and will explicitly qualify technical content in our articles.
If you’re interested in submitting an article for review, please contact our editorial staff. We aim to be timely in our review process and will provide feedback as quickly as possible.
Thanks for reading— we look forward to sharing advances in machine learning applications within the industry.